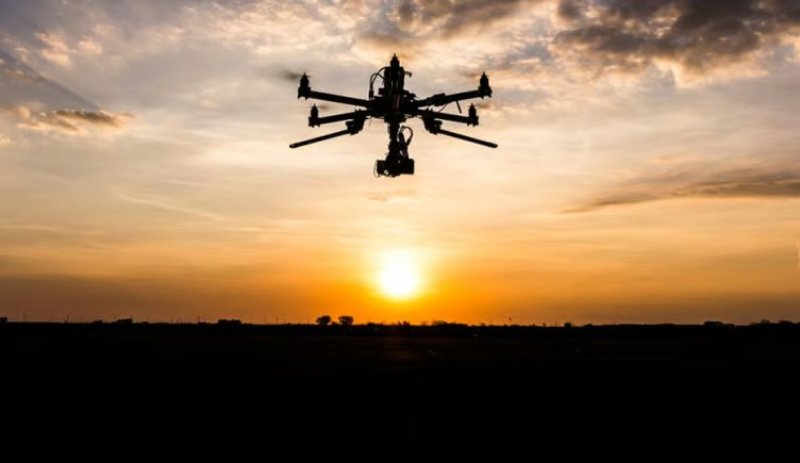
This Startup Is Developing AI Capable of Autonomous Drone Flight and Decision-making
- Business
- March 21, 2024
What precisely qualifies as human intellect and whether a machine’s functioning may be sufficiently akin to that of a human brain are key to the discussion of artificial intelligence’s potential limits.
Stanhope AI, a UK-based company, is developing its models based on neuroscience principles and drawing inspiration from the predictive, hierarchical machinery found in human brains, rather than aiming for artificial general intelligence (AGI).
An AI that doesn’t require training is the end product. All it really needs to know is that it exists, be given a preexisting belief system, and then take off (literally) into the actual world, utilizing sensors to gather information from its surrounds. Similar to how you update (or reinforce) your worldview as you see, hear, and feel new information that broadens your understanding.
The University College London spinout company has secured £2.3 million for its “agentic AI,” which is influenced by neuroscience. We spoke with Rosalyn Moran, a professor of computational neuroscience and co-founder and CEO of the firm, to find out more about its technology and long-term goals.
Stanhope’s AI’s Multilayered “brain”
The approach used by Stanhope AI is based on the idea that the brain maintains a model of the world and is always looking for new data to update and confirm it.
According to Moran, “the AI has a ‘brain’ a few levels deep, and its sensors are at the very bottom of the brain.” Here, the sensors are cameras and LiDAR, which represent our eyes, respectively.
“And then those feed into a predictive layer that will try and say, ‘Okay, I saw a wall over there. Now I don’t need to keep looking’. And it’s built into a more interesting cognitive prediction at the higher levels. So it’s very much like a hierarchical brain.”
Since the brain is our most energy-demanding organ, it makes predictions similar to this one in order to make sense of the world and conserve energy. This is a concept from neuroscience known as “active inference,” which is a component of the Free Energy Theory, which was created by Karl Friston, a theoretical neurobiology professor and co-founder of Moran.
“I don’t need to check every pixel on the wall to make sure it’s a wall — I can fill in a bit. So that’s why we think the human brain is so efficient,” Moran adds.
In the interest of energy efficiency, your perception of the environment is essentially a function of how your brain anticipates you will see it. To our brains’ credit, however, those predictions are then improved upon in response to sensory input. The model developed by Stanhope AI operates similarly, utilizing visual cues from its surroundings. On the basis of the updated, real-time data, it then takes judgments on its own.
Large Training Data Sets Are Not Necessary
This approach of AI is very different from more conventional machine learning techniques, like training LLMs, which are limited to the data that their trainers can supply.
“We don’t train [our model],” Moran says. “The heavy lifting is done in establishing the generative model, and making sure that it is correct and has consistent priors with where you might want it to operate.”
All of this is exciting in theory, but practical applications are necessary for a firm to emerge beyond the lab. According to Stanhope AI, their AI can run on self-governing devices like robots and delivery drones. The Federal Agency for Disruptive Innovation in Germany and the Royal Navy are among the partners testing the technology on drones at the moment.
Scaling from smaller models operating in lab settings to larger ones that can learn to navigate a much more expansive landscape has proven to be the startup’s biggest technological hurdle to date.
“In order to construct much larger worlds for our drones, we had to use three mathematical routes to do much more efficient free energy calculations,” says Moran. She also notes that a major engineering challenge was locating the appropriate hardware that the business could access and manage on its own, independent of outside vendors.
Agentic AI’s latest wave
The company claims that Stanhope AI’s “Active Inference Models” are fully autonomous and have the ability to improve and rework its forecasts. This is a component of a new phase of “agentic AI,” which constantly learns from differences between predictions and real-time data in an attempt to “guess what will happen next,” much like the human brain does. Significant (and costly) prior training is not required, and the method significantly reduces the possibility of AI “hallucinations.”
White box models, with “explainability built into its architecture,” are a notable feature of Stanhope’s AI. “We make sure that it’s working absolutely perfectly in simulation,” adds Moran. If the drone or AI behaves strangely, we investigate its beliefs and the reasons for its actions. Thus, it’s a totally distinct approach to AI development. According to her, the goal is to increase the influence of robotics and artificial intelligence in practical situations by transforming their capacities.
The £2.3mn fundraising round for Stanhope AI was led by the UCL Technology Fund. Along with a number of industry investors, the Creator Fund, MMC Ventures, Moonfire Ventures, and Rockmount Capital also took part.
Professor Rosalyn Moran, Director Professor Karl Friston, and Technical Advisor Dr. Biswa Sengupta launched Stanhope AI in 2021.